Network Analysis and Graph Machine Learning
- 2-2 ECTS credits
- Academic year 2024-2025
- DP00AY84
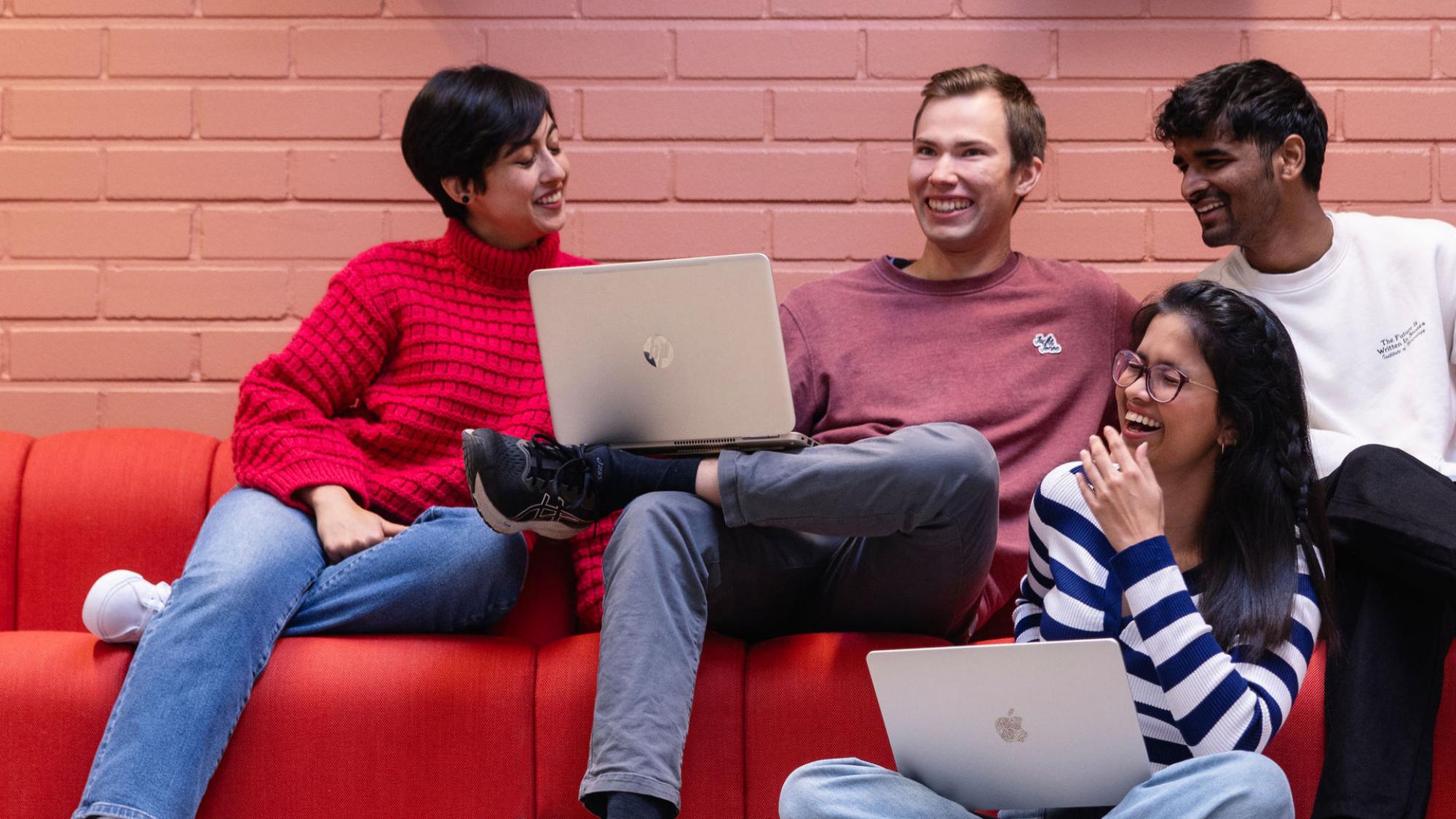
Education information
Implementation date
19.05.2025 - 23.05.2025
Enrollment period
-
Education type
Field-specific studies
Alternativity of education
Optional
Location
Linnanmaa
Enrollment and further information
Contact Lauri Lovén (lauri.loven@oulu.fi) for enrollment issues if there are any.
Education description
- Contact teaching hours = 10 hour (Given by Prof. Joydeep Chandra)
- Contact exercise hours = 7.5 hour (coding sessions managed jointly by Prof. Joydeep Chandra and Shruti Saxena)
- Independent self-studies = 10 hour (Slides and Reference materials to be provided beforehand, which should be looked into before the class)
- Lab work and assignments = 26.5 hour
Many real-world systems can be modeled as networks of interconnected entities, where the number of entities can be vast, forming large-scale networks. Examples include knowledge graph entities, keyword co-occurrence graphs in natural language, user interaction graphs in social networks, protein-protein interaction networks, Internet router networks, IoT edge devices, and financial transaction networks. Analyzing these networks is essential for relational learning tasks and creating frameworks that capture the intrinsic structure of data. This course will primarily cover methods for studying the properties of such large networks and applying advanced machine-learning techniques to these networks for various downstream applications.
By the end of the course, participants are expected to achieve the following outcomes:
- Represent and analyze graph structures effectively.
- Identify significant and recurring patterns in data using network motifs and community structures.
- Understand and apply traditional machine learning techniques to graph data.
- Utilize graph-structured data to enhance predictive capabilities through graph neural networks.
Course Contents:
The course will cover the following topics:
- Fundamentals of graphs and essential background knowledge
- Characterization of graph properties, including measures and metrics
- Basics of spectral graph analysis and its applications, such as graph partitioning and community detection
- Overview of machine learning applications on graphs, including shallow and deep learning techniques for generating node, link, and graph embeddings
- Use cases of graph neural networks across various domains