Sensing and deep learning in increasingly complex environments
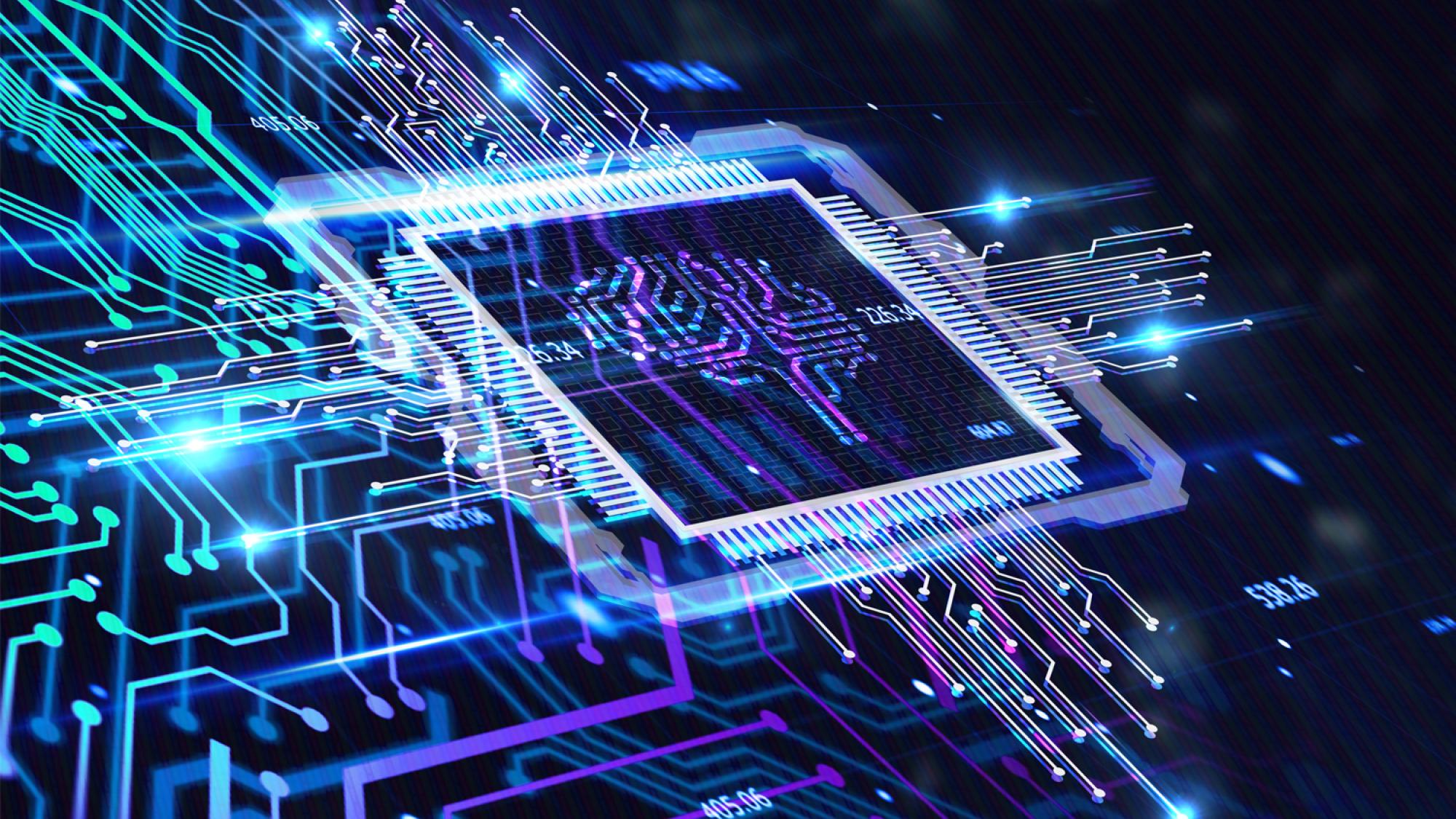
In Praneeth Susarla’s research, an antenna adopts the role of a sensor that transmits and receives radio signals. He aims to efficiently integrate sensor functionalities such as MIMO and massive MIMO using the framework of reinforcement learning over radio beamforming communications.
“Integrated artificial intelligence like reinforcement learning with millimeter wave radio beamforming has high massive potential in parallel with the development of millimeter wave radios for sensing besides communications,” Susarla underlines.
Now, he is developing a learning framework using Reinforcement Learning that can perform beamforming in an online manner under generic channel conditions. He is also contributing to the development of using mmWave radios for sensing besides communications in 5G and beyond networks. This involves integrating learning-based activity detection algorithms with 5G and 6G radio hardware.
“Using radios for sensing besides communications could have a significant impact in the society and can provide good business opportunities in the future,” Susarla says. “This enhancement of radio capabilities can be well integrated with other sensors such as Camera and Lidar. It can also be disruptive in many applications such as human activity detection, vital sign monitoring, and advanced analytics based on people’s presence.”
Mastering our arbitrary 3D environment
While deep learning has been successfully used to solve various 2D computer vision problems, the major challenge of computer vision is that our surrounding world is essentially three-dimensional. It consists of an unorganized set of arbitrarily shaped and positioned 3D objects with appearance that can change drastically due to geometric and photometric variations such as 3D rotations, shading and partial occlusions.
Nowadays 3D computer vision combines methods from conventional geometric vision and modern deep learning. “Our research covers various aspects of 3D imaging, where cameras are used for acquiring 3D information from the environment,” Professor Janne Heikkilä describes. “For example, we have carried out pioneering work on geometric camera calibration and published one of the standard algorithms for RGB cameras that has been used extensively both in industry and academia.”
The team’s recent works include, for example, RGBD (+depth) camera calibration, a SLAM system called DT-SLAM, and methods for dealing with motion blur in mobile photography.
“One research problem that we are currently investigating, is how to extract depth information from a single RGB image,” Heikkilä says. “Another ongoing research line aims to the development of novel view synthesis methods that enable rendering images of 3D environment from arbitrary viewpoints based on a small set of reference images.”
The methods that Heikkilä and his team develop are generic and they can be used in various applications where 3D perception, localization, mapping, and navigation are needed. Key application areas include, for example, mobile imaging, augmented and virtual reality, and robotics.
Cutting the energy demands
With the nearly endless amount of possible application areas, one key challenge arises. The training and inference of deep convolutional neural networks, as the major machine learning technique, requires tremendous energy supplies. Cutting the energy demands saves huge amounts of energy and environmental costs and calls for improved solutions - across layers.
In his research, Mehdi Safarpour combines techniques from the transistor level up to architecture and algorithm levels as he attacks the problem of energy efficiency in digital accelerators, through a holistic approach. He targets the problem of tremendous increase in energy demands of emerging technologies especially in deep neural networks and massive MIMO scenarios.
“As an 6G-oriented example, take massive MIMO antennas. With the increase in number of antennas of a MIMO application, the number of arithmetic operations increases dramatically,” Safarpour asserts.
Most of the algorithms for MIMO heavily involve matrix computations, which happen to be the most compute intensive parts of MIMO algorithms. “We have created a low-power solution, which is specific for general matrix operations,” Safarpour says. “According to our experiments, the energy for matrix computations can be reduced 50% without any performance loss. We perceive that our solution can be incorporated in any other applications which require lots of matrix operation and which are restricted in power or energy budget.”
He is now working on reducing power through reducing voltage. “We have developed a couple of solutions that enable reducing voltage of e.g., a GPU or an FPGA device that is running a computationally intensive algorithm without affecting the end results,” Safarpour says. “This is exciting as it means we can do more processing with less energy consumption. We have demonstrated two times higher energy efficiency with trivial trade-offs using commercial FPGAs.”
However, reducing voltage leads to errors in computations. To tackle this challenge, Safarpour and colleagues have developed a method that can detect and tackle those hardware errors and make the system recover from failure. “We have shown 50% savings in common matrix applications by experimentation on a FPGA device with ~ZERO decrease in reliability or system performance,” Safarpour summarizes.
The developed methods apply widely to challenges in machine learning accelerators and communication systems and include numerous benefits. They are easy and cheap to implement regarding the time investment and they can be integrated with even High-Level-Synthesis tools.
At the same time, the methods produce multiple folds power consumption savings on the FPGA and ASIC that are used for computations of MIMO and Deep Learning algorithms. They also increase in reliability of their processing platforms. “Even if the voltage is not intended to be scaled down, our low overhead method adds to reliability of computations,” Safarpour says. “This can for instance be applied to satellite communications.”
Futhermore, the methods relax thermal issues of the FPGAs, e.g., in base-stations, by operating in Inverse Temperature Dependence region. “We have been able to show how heat enables faster clocking at lower voltages and our method is cable of exploiting that,” Safarpour concludes. And with smaller cell sizes in the future, leading to a massive rise in the number of base stations, this may well be a winning solution.
More than 200 researchers work around different research topics related to artificial intelligence (AI) at the University of Oulu. The first AI research group – Machine Vision Group, the predecessor of Center for Machine Vision and Signal Analysis - (CMVS), was established as early as in 1981. The research truly has long roots.
Artificial Intelligence research and development at the University of Oulu covers a wide range of different areas: computer vision, emotion AI, machine learning, robotics, edge computing, and medical, industrial and atmospheric applications of AI methods.
“Throughout the years, many spin-off companies related to AI have been born,” says Olli Silvén, the head of the CMVS research unit. “Our group’s long-term expertise has a lot to offer for 6G development as well.”