6G Cure: Robust threat prevention for AI/ML for beyond 5G and 6G
6G Cure
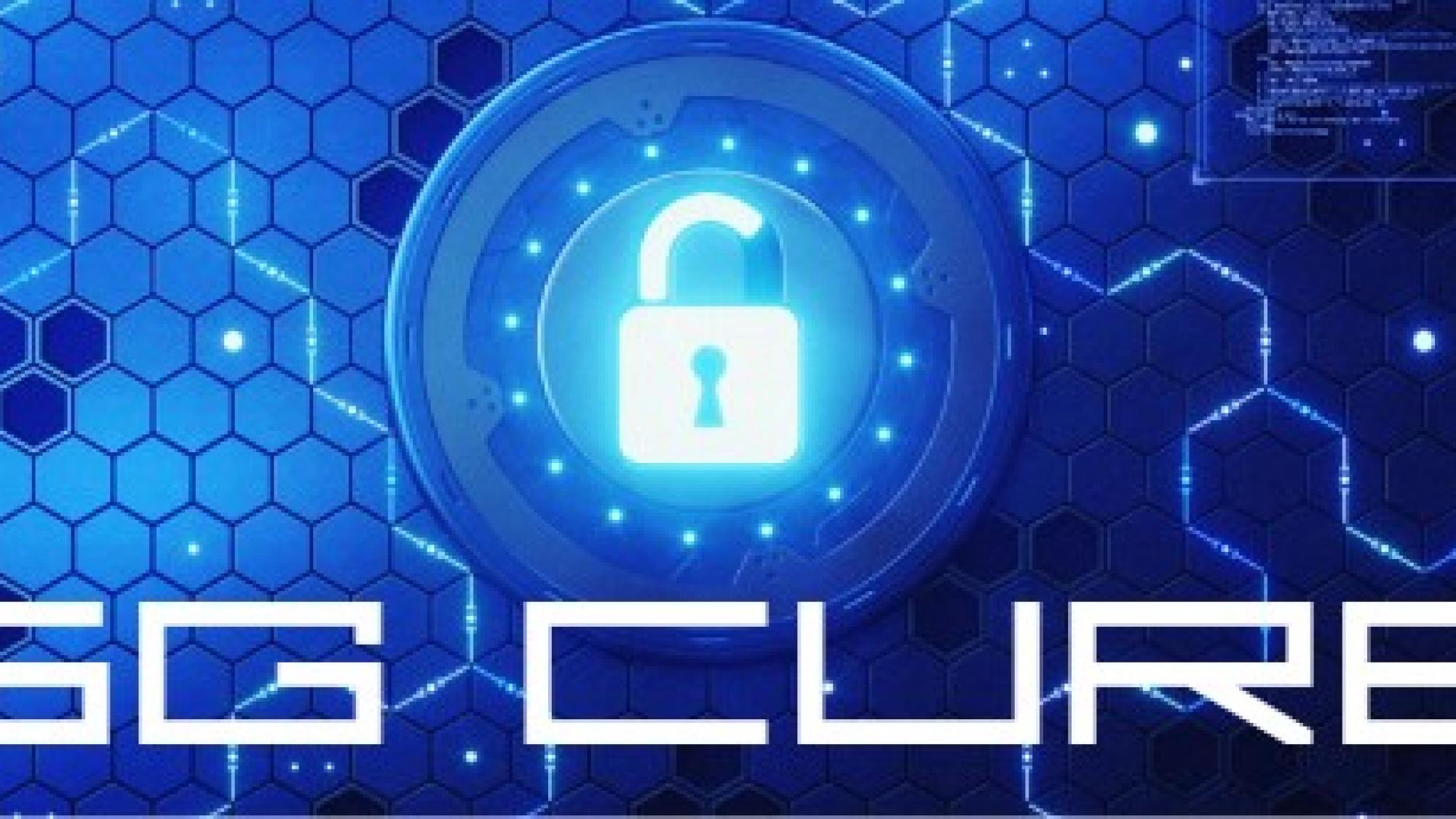
Project information
Project duration
-
Funded by
Business Finland
Funding amount
553 000 EUR
Project coordinator
University of Oulu
Contact information
Project leader
- Professor
Other persons
- Doctoral Researcher
- Doctoral Researcher
Researchers
Project description
5G Advanced and 6G networks and systems will include an increased amount of distributed ML/AI algorithms implemented to various network functions both in radio access and core networks. The used algorithms may not be designed to be robust against certain types of attacks such as model poisoning and inference attacks. Poisoning attacks launched by adversaries focus on decreasing the quality of the AI/ML models while inference attacks try to reconstruct the data that the AI/ML algorithms were trained. These kinds of attacks can decrease the system performance regarding the KPIs, and may also result in increased system downtime and service unavailability in critical applications.
The 6G Cure project proposes robust techniques for Machine Learning (ML), that eliminate the adversaries in the local network and provides early detection, prevention, and mitigation of poisoning attacks and membership inference attacks on decentralized ML models. Proposed robust algorithms consider different architectures of learning networks, remove the malicious model updates received from the peer users and consider only the legitimate update to continue the learning process. When the data is not visible to peers in decentralized learning, only the properties of the model update can be used to identify the malicious nodes in the system. In “Gossip Learning”, partial model updates may be shared to minimize the risk of membership inference attacks. This makes the implementation of robust algorithms even more challenging in the presence of poisoning and membership inference attacks as the defender has less data for the analysis.
These robust techniques are applicable in various 5G Advanced and 6G use cases including Unmanned Aerial Vehicle (UAV) networks, self-driving cars, critical medical and industrial applications, and automated network management. The proposed solutions can be implemented for example as part of advanced network slicing solutions in 5G Advanced and 6G.
Project results
International peer-reviewed journal articles
- Y. Siriwardhana, P. Porambage, M Liyanage, S Marchal, M Ylianttila, " SHIELD - Secure Aggregation against Poisoning in Hierarchical Federated Learning," accepted to IEEE Transactions on Dependable and Secure Computing (JuFO-3, Impact factor 7.0). https://ieeexplore.ieee.org/document/10704049
International peer-reviewed conference proceedings
- P. Munaweera, S. Prasad, T. Hewa, Y. Siriwardhana, M. Ylianttila,"Federated Learning-powered DDoS Attack Detection for Securing Cyber Physical Systems in 5G and Beyond Networks", 14th International Conference on the Internet of Things (IoT 2024), November 19--22, 2024, Oulu, Finland, ACM ISBN 979-8-4007-1285-2/24/11, https://doi.org/10.1145/3703790.3703822
- J. Kehelwala, Y. Siriwardhana, T. Hewa, M. Liyanage, M. Ylianttila, "Decentralized Learning for 6G Security: Open Issues and Future Directions", accepted to EuCNC & 6G Summit, 3-6 June, 2024. Antwerp, Belgium. https://www.eucnc.eu/
- Y. Siriwardhana, P. Porambage, M. Liyanage, S. Marchal and M. Ylianttila, "Robust Aggregation Technique Against Poisoning Attacks in Multi-Stage Federated Learning Applications," in the Proceedings of 2024 IEEE 21st Consumer Communications & Networking Conference (CCNC), Las Vegas, NV, USA, 2024, pp. 956-962.
- T. Hewa, P. Porambage, M. Liyanage, M. Ylianttila," Towards Attack Resistant Federated Learning with Blockchain in 5G and Beyond Networks", 2023 EuCNC & 6G Summit - Posters.
Demonstrations
- Y. Siriwardhana, P. Porambage, M Liyanage, S Marchal, M Ylianttila ,“Defending against Poisoning Attacks in Hierarchical Federated Learning Systems in 6G Networks”, accepted to Brooklyn 6G Summit 2024. https://b6gs.com/agenda2024/
- S. Prasad, P. Munaweera, T. Hewa, Y. Siriwardhana, M Ylianttila, " Simulation of IIoT-Driven Attack Vectors on 5G Core Networks: Dataset Generation and Machine Learning Based Detection ", acceptedto 14th International Conference on the Internet of Things (IoT 2024). https://iot-conference.org/iot2024/
- J. Kehelwala, Y. Siriwardhana, T. Hewa, M. Ylianttila, "Defending against poisoning attacks in Federated Learning systems in autonomous driving", Brooklyn 6G Summit, B6GS 2023 – Demo.
Datasets
- S. Samarakoon, Y. Siriwardhana, P. Porambage, M. Liyanage, S. Chang, Jinoh Kim, Jonghyun Kim, M. Ylianttila, "5G-NIDD: A Comprehensive Network Intrusion Detection Dataset Generated over 5G Wireless Network". arXiv:2212.01298 [cs.CR],https://doi.org/10.48550/arXiv.2212.01298 (Pre-print of ongoing work)