Data Mining and Remote Sensing Techniques in Hydrological Modeling
DMARS
Funders
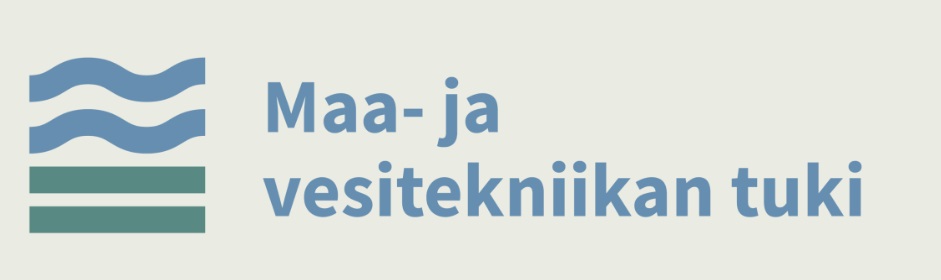
Project information
Project duration
-
Funded by
Other Finnish
Project funder
Project coordinator
University of Oulu
Unit and faculty
Contact information
Project leader
- Associate Professor (Tenure Track)
Project description
The proposed project aims to leverage innovative data mining and remote sensing techniques for modeling hydrological processes in various global case studies. The primary goal is to enhance water resource management by applying cutting-edge data mining methods to address water shortages, overcome challenges associated with data unavailability, and monitor the River Ice Phenomenon. Water scarcity and the lack of sufficient data are significant hurdles in effective water resource management, particularly in the realm of hydrological modelling.
Project actions
The project was initiated to address the critical challenges in water resource management, underscored by the urgency to tackle water shortages, data inaccessibility, and the impacts of climate change on hydrological processes. It aimed to exploit novel data mining and remote sensing techniques to provide comprehensive solutions, rooted in collaborative efforts among hydrology, remote sensing, and data science experts. These experts identified an essential need for advanced technological approaches to understand and mitigate global-scale challenges.
Regarding the achievements of the project's original goals, we have made notable progress. The substantial funding received enhanced our team's expertise in machine learning, data mining, and remote sensing, allowing us to apply our methodologies worldwide, including in Finland, Norway, Turkey, and Iran. The project also supported the salaries of two doctoral researchers at the University of Oulu, further contributing to our team's capacity.
Innovative data mining techniques led to new insights into water shortages, improving effective water resource management strategies. Key accomplishments include:
- Developing an approach for mapping suitable sites for Rainwater Harvesting (RWH) structures using socio-environmental variables and artificial intelligence algorithms, addressing water scarcity.
- Utilizing hybrid evolutionary algorithms to estimate Groundwater Level (GWL) fluctuations more accurately, aiding in integrated water resource management.
- Introducing a methodology, in collaboration with Turkish scholars, for predicting sea level changes, crucial for coastal structure design and harbor operations.
- Proposing a new hybrid Random Forest model for drought forecasting, enhancing the accuracy of drought index predictions.
- Advancing flood and flow prediction with a new evolutionary time series model and a hybridized model based on neural networks and swarm intelligence for flood-inundation mapping.
- Addressing ecohydrological modeling challenges by proposing jittered binary genetic programming to identify dominant parameters affecting Arctic charr occurrence.
The application of remote sensing technology and satellite imagery has been pivotal in overcoming data limitations, enhancing our understanding of hydrological processes worldwide. Notably, we utilized the Google Earth Engine extensively for water resource engineering applications, producing detailed analyses of surface water distribution, evapotranspiration, and land cover, among others. This approach enabled us to assess vegetation health, sea surface salinity, and river ice cover with high spatial resolution, offering new perspectives on river hydrology and the effects of extreme flow events.
In summary, the project has not only met but exceeded many of its original objectives, providing innovative solutions to pressing water management challenges and fostering significant advancements in hydrological modeling and environmental monitoring.
Project results
The Project has facilitated a prolific output of scholarly work, significantly contributing to the field of hydrology and water resource management. Here is a summary of the main results and products developed through this project:
Journal Articles
Efficient Rainwater Harvesting Planning
Darabi, H., Moradi, E., Davudirad, A.A., Ehteram, M., Cerda, A. and Haghighi, A.T. (2021). Journal of Cleaner Production, 311, p.127706.
https://doi.org/10.1016/j.jclepro.2021.127706
Groundwater Level Modeling Using Multiobjective Optimization with Hybrid Artificial Intelligence Methods
Banadkooki, F.B. and Haghighi, A.T. (2023). Environmental Modeling & Assessment.
https://doi.org/10.1007/s10666-023-09938-6
Sea Level Prediction Using Machine Learning
Tur, R.; Tas, E.; Haghighi, A.T.; Mehr, A.D. (2021). Water, 13, 3566.
https://doi.org/10.3390/w13243566
A New Evolutionary Hybrid Random Forest Model for SPEI Forecasting
Danandeh Mehr, A., et al. (2021). Water, 14(5), 755.
https://doi.org/10.3390/w14050755
A New Evolutionary Time Series Model for Streamflow Forecasting in Boreal Lake-River Systems
Dnandeh Mehr, A., et al. (2022). Theoretical and Applied Climatology, 148(1-2).
https://doi.org/10.1007/s00704-022-03939-3
A Hybridized Model Based on Neural Network and Swarm Intelligence-Grey Wolf Algorithm for Spatial Prediction of Urban Flood-Inundation
Darabi, H., et al. (2021). Journal of Hydrology, 603, 126854.
https://doi.org/10.1016/j.jhydrol.2021.126854
Factors Affecting the Presence of Arctic Charr in Streams
Danandeh Mehr, A., et al. (2022). Ecological Indicators, 142, 109203.
https://doi.org/10.1016/j.ecolind.2022.109203
Causes & Effects of Upstream-Downstream Flow Regime Alteration
Baubekova, A., et al. (2023). Science of The Total Environment, 858, 160045.
https://doi.org/10.1016/j.scitotenv.2022.160045
Environmental Flow Assessment for Intermittent Rivers Supporting the Most Poleward Mangroves
Baubekova, A., et al. (2024). Science of The Total Environment, 907, 167981.
https://doi.org/10.1016/j.scitotenv.2023.167981
Manuscript Submitted to Environmental Monitoring and Assessment Journal: Ice Phenology in the Arctic: Case Study River Tornionjoki
Doctoral Theses
Catchment-Estuary-Coastal Systems Under Climate Change and Anthropogenic Pressure
Baubekova, A. (2023).
https://urn.fi/URN:ISBN:9789526238005
Past, Present, and Future of River Flow Regime in Nordic Region Focusing on River Ice Break-Up Events
Jalali Shahrood, A. (2023).
https://urn.fi/URN:ISBN:9789526238722