NEW SOLUTIONS FOR DATA ASSIMILATION AND COMMUNICATION TO IMPROVE HYDROLOGICAL MODELLING AND FORECASTING
WATERLINE
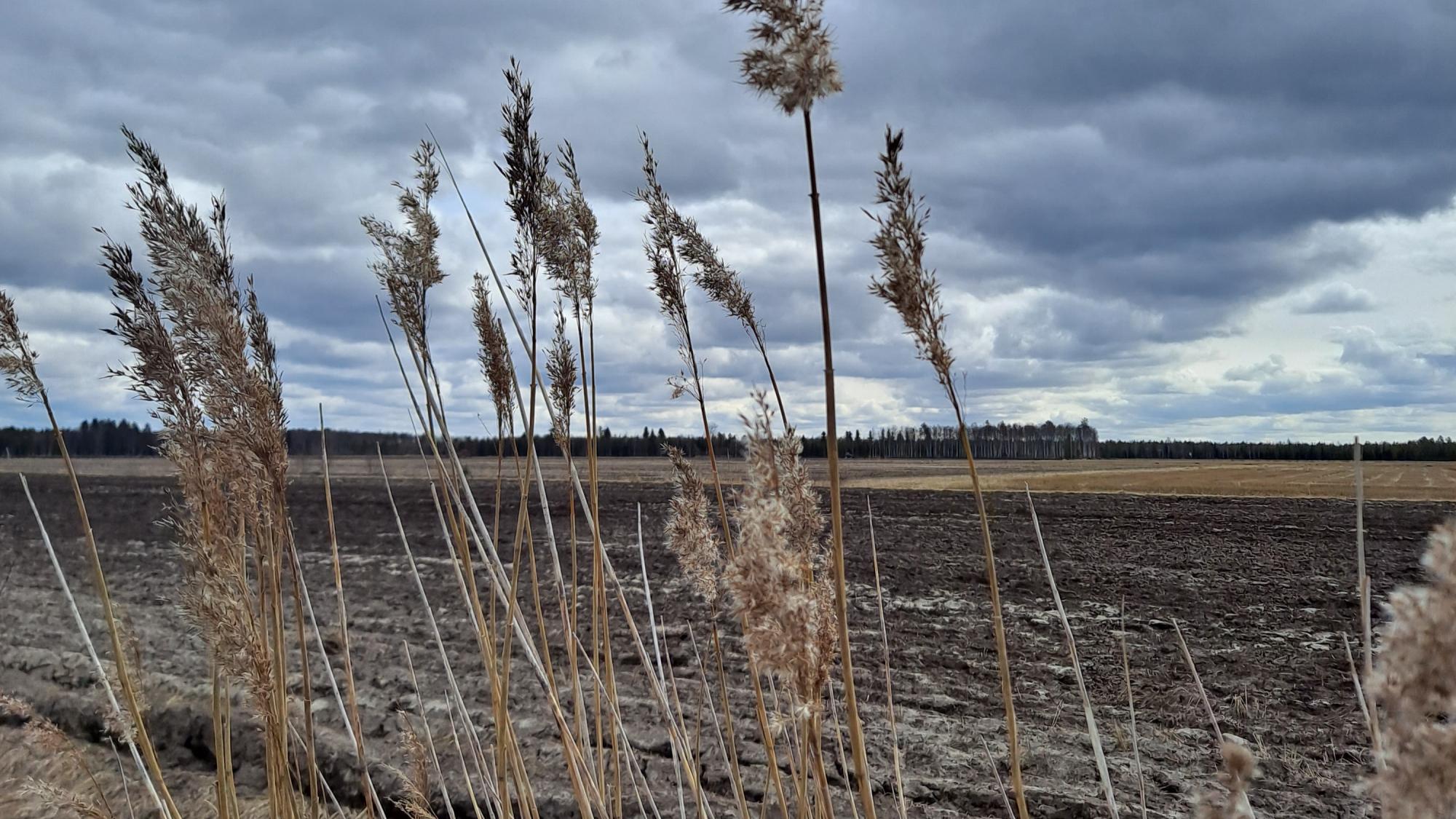
Project information
Project duration
-
Funded by
Research Council of Finland
Funding amount
449 595 EUR
Project coordinator
Environmental Engineering Department, Democritus University of Thrace, Greece
Unit and faculty
Researchers
Project description
Hydrological models are essential tools in water resources assessment and management. Advanced computational algorithms can
simulate the relevant physical processes and form the feedback mechanism across a wide range of spatial and temporal scales.
However, a bottleneck of these models is the lack of environmental observations to calibrate model parameters and to assess the
robustness of model predictions. WATERLINE will employ multi-source information from remote sensing, historical data, in-situ data from meteorological networks as well as crowdsourced measurements to improve hydrological models and their predictions. The
relevant physical processes and heterogeneity of hydrological catchments need to be integrated in hydrological models as a basis for reliable model predictions. A major challenge in this endeavour is identifying the observation data with the highest information content to constrain model parameters. Unfortunately, neither in-situ networks nor remote sensing alone can provide sufficient information to capture the high spatial and temporal variability of hydrological processes. Recently, downscaling frameworks have been developed, building robust models between coarse scale products and high-resolution covariates using in-situ measurements. The lack of in-situ measurements to train such models can be overcome by the growing availability of crowdsourced observations. WATERLINE will
improve the efficiency and robustness of hydrological models through strategic integration of variables covering different spatial and temporal scales. Furthermore, we will optimize the computational performances to provide near real-time and short-term predictions of various hydrological states with unprecedented spatial detail. Improved representation of soil moisture, groundwater levels and recharge, stream discharge, and evapotranspiration can significantly advance the sustainable management of water resources for a wide range of stakeholders.