Data Analysis and Inference Group
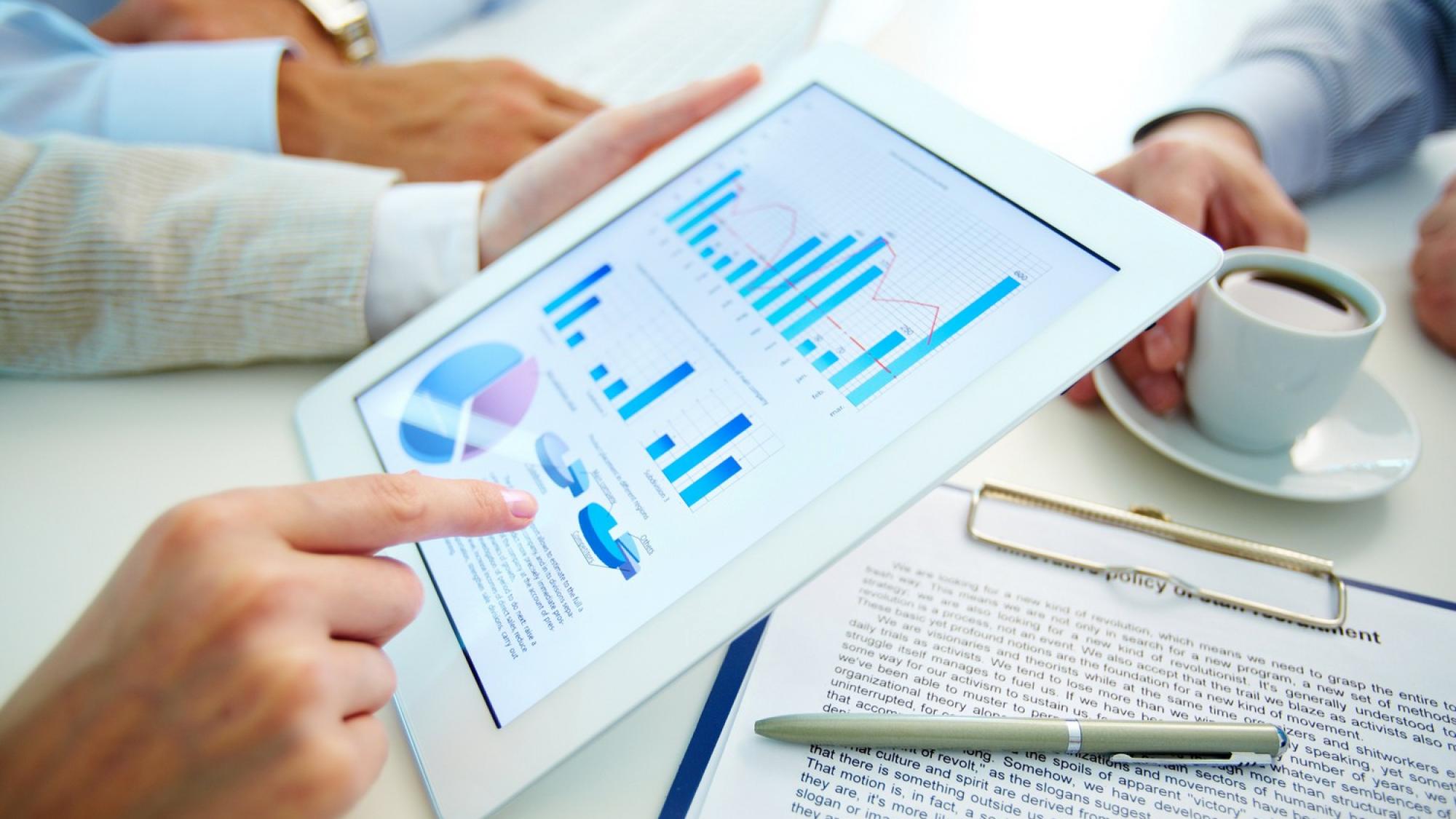
Research group information
Contact information
Research group leader
- Professor
Researchers
Research group description
Data Analysis and Inference Group conducts high-quality research in the field of computational data analysis and develops methods for diverse real-world modeling problems. Our strengths are a strong, versatile background in engineering, mathematics, statistics, and broad knowledge within application areas.
The research in DataAI is focused into interacting areas of artificial intelligence, big data, machine learning, industrial statistics and e-health.
Application areas include (e.g.):
- Activity monitoring
- Wearable sensors
- Intelligent environments
- Steel manufacturing
- Cognitive load assessment
- Quality improvement
- Context recognition
- Human-robot interaction
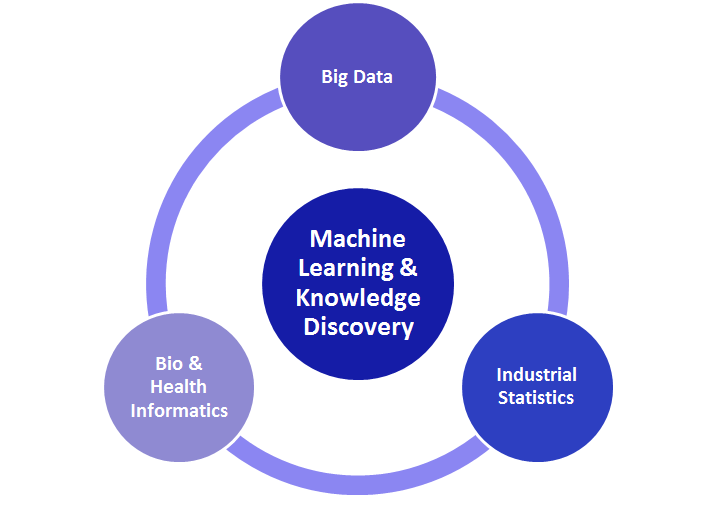
Big data
DataAI group has a long history in data analysis research. The application areas have varied from steel manufacturing and spot welding to human activity recognition from e.g. accelerometer data, and many others. Currently DataAI is taking steps to broaden its research area to include big data related problems as we see huge potential in this area. The potential is also recognized in reports that try to forecast the future key technologies.
Industrial Statistics
In the research on industrial statistics, data analysis approaches are developed for optimization, control and planning of industrial processes. The scope of applications has varied from spot welding to cheese manufacturing, and much of the research has focused on improving product quality in steel industry.
Machine Learning and Knowledge Discovery
Machine learning as a sub-field of artificial intelligence studies methods that can automatically detect patterns in data by optimizing the performance criteria from the examples or past experiments. A learned model can be used to predict the response variables or other properties of unknown patters. In our research, we are concentrating on the development of statistical (and probabilistic) learning methods which are able to tackle the different uncertainties and non-linear dependencies typically involved in the patterns and large-scale datasets. Furthermore, we are interested in particular algorithms which are able to utilize multi-modal and structured data in different data analysis and prediction scenarios such as classification, regression, clustering, and sequential modeling, using tools such as instance-based learners, neural networks, kernel methods, Bayesian non-parametric methods, and Bayesian filtering.